13 Spatial Data and Maps
Focus on rosm throughout the chapter.
Spatial data is central to many of our tasks as Data Scientists. Identifying spatial patterns and spatial correlations and relationships between those patterns offers opportunities for identifying, for example, locations of new services for populations. Imagine predicting common routes for travellers this morning, and dynamically routing public transport to meet those needs. Fundamental to the Data Scientist is the ability to process, visualize and then model spatial data. Done right maps can be a very effective communications tool. Numerous R packages work together to bring us a sophisticated mapping and spatial analysis capability.
The required packages for this chapter include:
Spatial data is central to many of our tasks as Data Scientists. Identifying patterns, correlations and relationships between those patterns delivers opportunities for delivering new services. Imagine predicting common routes for travellers this morning, and dynamically routing public transport to meet those needs. Fundamental to the Data Scientist is the ability to process, visualize and then model spatial data. Done right maps can be a very effective communications tool. Numerous R packages work together to bring us a sophisticated mapping and spatial analysis capability.
Your donation will support ongoing availability and give you access to the PDF version of this book. Desktop Survival Guides include Data Science, GNU/Linux, and MLHub. Books available on Amazon include Data Mining with Rattle and Essentials of Data Science. Popular open source software includes rattle, wajig, and mlhub. Hosted by Togaware, a pioneer of free and open source software since 1984. Copyright © 1995-2022 Graham.Williams@togaware.com Creative Commons Attribution-ShareAlike 4.0
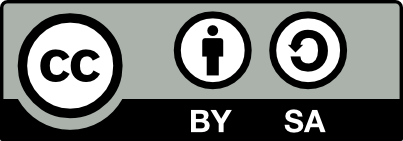