3 R Constructs
20210103 Here we introduce the language constructs of R including the powerful concept of pipes as a foundation for building sophisticated data processing pipelines in R.
To illustrate the R constructs we will take a copy of the
rattle::weatherAUS dataset (daily weather
observations from Australia) and save it as a variable named
ds
. The column names in the dataset are also normalised as
we would normally do as data scientists. The operations here will
become familiar as we progress through the book. To aid our
understanding for now though, we can ready the code as:
Obtain the weatherAUS dataset from the rattle package; clean up the column names using the clean_names function from the janitor package, choosing to align numerals to the right; save the resulting dataset into an R variable named ds.
For reference the resulting column names (variables) are:
## [1] "date" "location" "min_temp" "max_temp"
## [5] "rainfall" "evaporation" "sunshine" "wind_gust_dir"
## [9] "wind_gust_speed" "wind_dir_9am" "wind_dir_3pm" "wind_speed_9am"
## [13] "wind_speed_3pm" "humidity_9am" "humidity_3pm" "pressure_9am"
## [17] "pressure_3pm" "cloud_9am" "cloud_3pm" "temp_9am"
## [21] "temp_3pm" "rain_today" "risk_mm" "rain_tomorrow"
Your donation will support ongoing availability and give you access to the PDF version of this book. Desktop Survival Guides include Data Science, GNU/Linux, and MLHub. Books available on Amazon include Data Mining with Rattle and Essentials of Data Science. Popular open source software includes rattle, wajig, and mlhub. Hosted by Togaware, a pioneer of free and open source software since 1984. Copyright © 1995-2022 Graham.Williams@togaware.com Creative Commons Attribution-ShareAlike 4.0
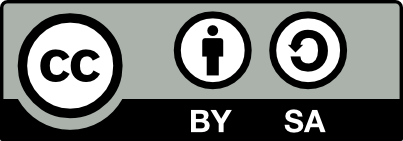