8.7 Modelling Roles
# Note the risk variable which measures the severity of the outcome.
risk <- "risk_mm"
# Note the identifiers.
id <- c("date", "location")
# Initialise ignored variables: identifiers.
ignore <- c(risk, id)
# Remove the variables to ignore.
vars <- setdiff(vars, ignore)
# Identify the input variables for modelling.
inputs <- setdiff(vars, target) %T>% print()
## [1] "rain_today" "temp_3pm" "temp_9am" "cloud_3pm"
## [5] "cloud_9am" "pressure_3pm" "pressure_9am" "humidity_3pm"
## [9] "humidity_9am" "wind_speed_3pm" "wind_speed_9am" "wind_dir_3pm"
## [13] "wind_dir_9am" "wind_gust_speed" "wind_gust_dir" "sunshine"
## [17] "evaporation" "rainfall" "max_temp" "min_temp"
# Also record them by indicies.
inputi <-
inputs %>%
sapply(function(x) which(x == names(ds)), USE.NAMES=FALSE) %T>%
print()
## [1] 22 21 20 19 18 17 16 15 14 13 12 11 10 9 8 7 6 5 4 3
Also create the formula for modelling. Note that the target variable is the final column of the dataset. The stats::formula() function treats the first column as the target so reverse the list here to automatically generate the correct default formula.
Your donation will support ongoing availability and give you access to the PDF version of this book. Desktop Survival Guides include Data Science, GNU/Linux, and MLHub. Books available on Amazon include Data Mining with Rattle and Essentials of Data Science. Popular open source software includes rattle, wajig, and mlhub. Hosted by Togaware, a pioneer of free and open source software since 1984. Copyright © 1995-2022 Graham.Williams@togaware.com Creative Commons Attribution-ShareAlike 4.0
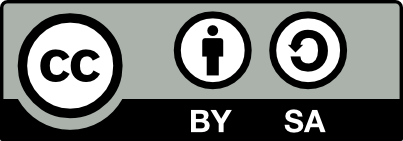