Preface
20210308
“The enjoyment of one’s tools is an essential ingredient of successful work.” Donald E. Knuth
This book is undergoing conversion from LaTeX to Bookdown. It is a work in progress and there remains numerous glitches. Please bear with us.
Welcome to the world of Artificial Intelligence (AI), Machine Learning, and Data Science. Data Science has come to describe many different activities that are driven by data utilising technological advances in AI and Machine Learning, amongst others. Indeed, we can think of Data Science as an ecosystem of both technology and experiences, shared through the freedom of open source software that implements tools for AI and Machine Learning. Open source software gives us the freedom to do as we want with the software, with very few, and ideally no, restrictions, except the requirement to maintain our freedoms.
The aim of this book is to gently guide the novice along the pathway to Data Science, from data processing through Machine Learning and to AI. I hope I can share the excitement of a fun and productive environment for exploring data with a focus on the R language as our platform of choice. R remains the most flexible and powerful language developed specifically for the analysis of data.
This book provides a guide to the many different regions of the R platform, with a focus on doing what is required of the Data Scientist. It is comprehensive, beginning with basic support for the novice Data Scientist, moving into recipes for the variety of analyses we may find ourselves needing.
On completing an install of R (which may take only a few minutes) you are ready to explore your data and beyond. All of the different types of data analyses are covered in this book, including basic data ingestion, data cleaning and wrangling, data visualisation, modelling and evaluation in order to discover new knowledge from our data. Tools for developers of systems to be deployed are well represented.
Your donation will support ongoing availability and give you access to the PDF version of this book. Desktop Survival Guides include Data Science, GNU/Linux, and MLHub. Books available on Amazon include Data Mining with Rattle and Essentials of Data Science. Popular open source software includes rattle, wajig, and mlhub. Hosted by Togaware, a pioneer of free and open source software since 1984. Copyright © 1995-2022 Graham.Williams@togaware.com Creative Commons Attribution-ShareAlike 4.0
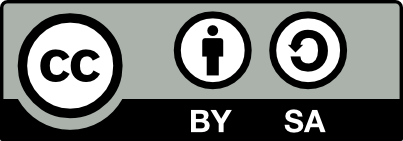